Grasping the need
Pfeiffer Vacuum has requested Phimeca to analyze the production data of its vacuum pumps. Two sets of data were available: on one hand, the sound power produced by each pump, and on the other, the metrological data of the parts forming each of these pumps.
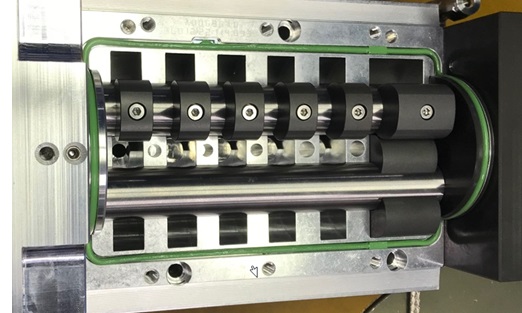
Data preparation
The variability of the sound power produced by the pumps is the root of a significant rate of non-compliance. Thus, it is important to analyse the sources of this variability. To do so, we have used the traceability of the parts forming each pump.
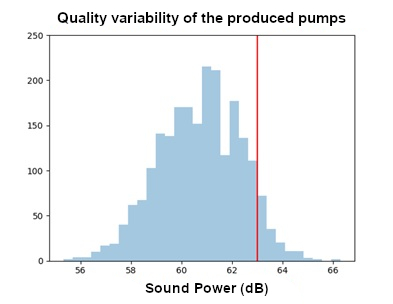
Predictive modelling
This project was realized within the Cloud Microsoft Azure platform. According to the customer’s needs, Phimeca makes use of different technologies, free or commercialized, outsourced or not, to model the information contained in the data.
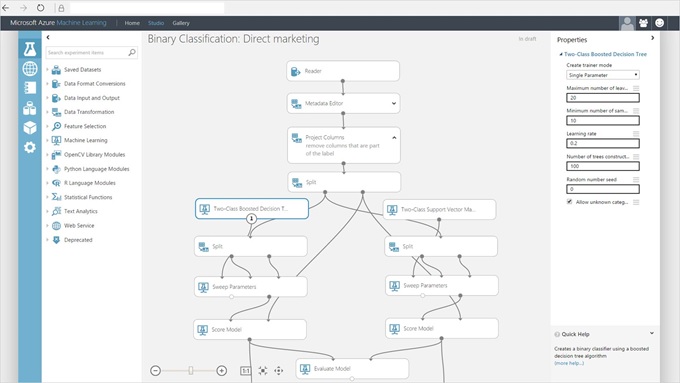
Added value
The data analysis has highlighted some unsuspected influential parameters among the several hundred parameters measured at the manufacturing outlet. Using a predictive model also allowed to divide by 2 the number of pumps we physically test, and thus, increase the production capacity.
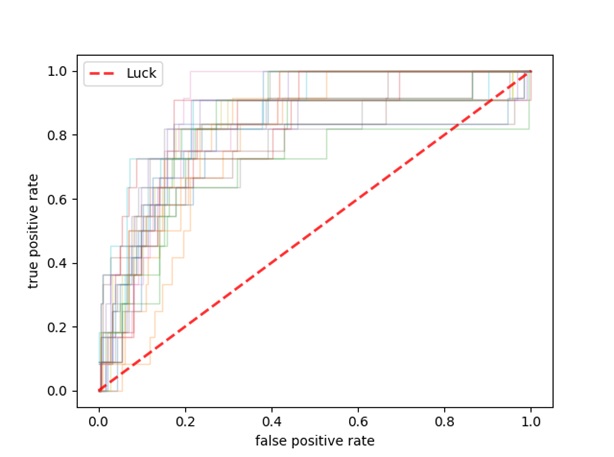
Intervention methodology
The artificial intelligence, as Phimeca conceived it, does not aim at replacing the experts, but at helping them in their decision-making situations and at giving more time for the higher added value tasks, economically and intellectually speaking. Furthermore, it adds up to a good understanding of the phenomena physics.
Discussion with the customer’s professional experts, review of the available data, identification of the need and establishment of a waybill.
Data appropriation, structuring, process of missing or abnormal data, standardization, sensitivity analysis, feature engineering, etc.
Regression models, classification models, neural networks, cross-validation
On-site reproduction, tool implementation, customer’s training, ROI measurement.